So why do we see these shifting, very different death estimates? Why is this hard to pin down? The number we are trying to establish here is the Case Fatality Rate, or CFR, and it's defined very simply as the number of deaths from a disease divided by the total number of people who have that disease: if 100 people get a disease and 10 of them die, that's a CFR of 10%. The math is a simple division, so what makes this hard to determine?
It turns out that there are two primary sources of uncertainty in calculating a CFR:
- Uncertainty in knowing how many people actually have the disease (the denominator of the percent).
- A timeline specific uncertainty in knowing how many deaths will occur (the numerator of the percent) that happens if you are trying to calculate a CFR in the middle of an epidemic.
I wanted to try to illustrate both of these problems with estimating the fatality rate for a new disease, so I came up with some scenarios and graphs that demonstrate them. You can look at all the numbers I came up with for this scenario on this google spreadsheet: https://docs.google.com/spreadsheets/d/1ePV5OnN5xeHYmXyzxbpccYUrxPnffTeU6_YtFOYmiLU/edit?usp=sharing
The Disease Timeline
The first thing I did was to generate some numbers in a spreadsheet for an infection in a location that behaves roughly as we have seen Covid-19 behave. My model for the infection curve that I generated was roughly South Korea, as it has the most complete data for a rise-and-fall of the disease so far. I generated about 3 1/2 months of infections-per-day numbers that rise exponentially for the first 30 days, then abruptly level off due to interventions, and then decay rather rapidly after a time. Then I assumed that some percentage of people with the disease would require hospitalization (10% is the amount I chose), but that on average, people wouldn't need hospitalization until they'd been infected for 10 days. Then I assumed that some percentage of people who were hospitalized (again, 10% is what I chose) would die, on an average of 7 days after hospitalization. This gives a total real-life CFR of 1%. It also give us three graphs which show the same curve shape, but scaled down and shifted in time for the hospitalizations and again for the deaths.
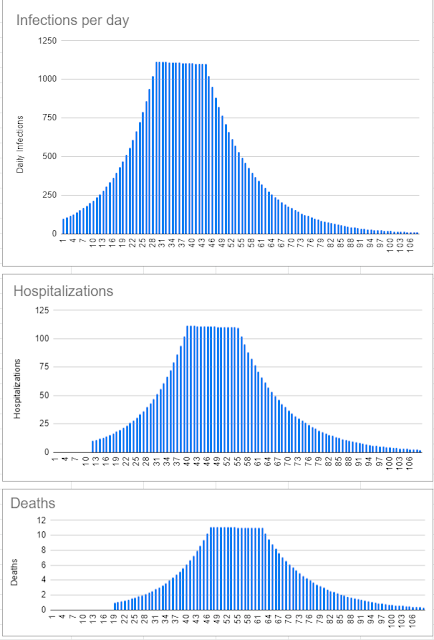
(The curve shapes are pretty terrible because I don't know how to do logistic curves in Google Spreadsheets, but this is fine to get the point across.)
What is the Apparent CFR?
With this timeline established, I then asked the question: given this disease progression timeline, what would the CFR appear to be at any given moment? At any moment, if the people in this scenario stopped to tally up all the deaths that had occurred so far and divide that by all the infections they knew about, what would they think the CFR was?
And the answer to this is, it depends on what infections they know about.
So let's look at three different scenarios:
- Poor knowledge of infections
- Consistently good knowledge of infections
- Perfect knowledge of infections.
In all three scenarios, we are going to assume that we know about all infections that become hospitalized, so these people always get counted in with the known infected. How many non-hospitalized infected are known is what varies for each scenario.
In the first scenario, we are going to assume that our sample nation was unprepared and did almost no testing in the general population until some amount of people started dying: call this the "Italian Paradigm". Even after testing starts, it ramps up slowly, only reaching full capacity by the end of the outbreak.
Furthermore, we are going to assume that there is a large body of infected people who have no symptoms and who never get tested--say, half of all the infected people. So in the "poor knowledge" scenario, the testing for non-hospitalized cases starts near zero and only goes up to a bit above 40% of total coverage at the best.
In the second scenario, we are going to assume that the sample nation was prepared and jumped on testing right away: call this the "South Korean" paradigm. Here we are going to assume a constant high rate of testing that catches most symptomatic infected people. However, we are still going to assume a large body of infected people who are asymptomatic who never get tested. So for this scenario, we are saying that 45% of all infected people outside of the hospital system are known about, as well as all the people within it.
In the third scenario, we are going to assume that we somehow magically know all of the infected people right away.
In the first scenario, we are going to assume that our sample nation was unprepared and did almost no testing in the general population until some amount of people started dying: call this the "Italian Paradigm". Even after testing starts, it ramps up slowly, only reaching full capacity by the end of the outbreak.
Furthermore, we are going to assume that there is a large body of infected people who have no symptoms and who never get tested--say, half of all the infected people. So in the "poor knowledge" scenario, the testing for non-hospitalized cases starts near zero and only goes up to a bit above 40% of total coverage at the best.
In the second scenario, we are going to assume that the sample nation was prepared and jumped on testing right away: call this the "South Korean" paradigm. Here we are going to assume a constant high rate of testing that catches most symptomatic infected people. However, we are still going to assume a large body of infected people who are asymptomatic who never get tested. So for this scenario, we are saying that 45% of all infected people outside of the hospital system are known about, as well as all the people within it.
In the third scenario, we are going to assume that we somehow magically know all of the infected people right away.
I generated the numbers of known infected people per day given these knowledge restrictions, for each scenario. Then a calculated what the apparent CFR would look like if it was calculated each day by taking the sum of deaths so far and dividing it by the sum of these known infected. Here's what I got:
Poor Knowledge of non-Hospital Infections
What we see here is that due to the lack of good knowledge of how many non-hospitalized infections there are, the apparent CFR almost immediately jumps up to an artificially high number. Given no extra-hospital testing, this would eventually rise to 10%, which is the fatality rate I chose for infections that get to the hospitalization stage. Once some testing starts to kick in, though, the number starts to go down, as knowledge of total infected starts to get better. However, while this does happen, more people continue to die, and this effect starts taking over and the apparent CFR starts rising again. It finally rests at a number 6 times what it should be, which indicates that while all deaths are counted by the end, only 1/6th of the total infected were ever counted.
I am also plotting on this chart (for comparison purposes) the third scenario, where we magically know all infections at all time: this is the red line on the graph. Note that even with perfect knowledge, due to the time lag of when people die, this also gives an incorrect apparent CFR up until the very end.
Consistently Good Knowledge of non-Hospital Infections
Here we see that due to prompt testing, we don't see an initial spike of the apparent CFR to unrealistic levels dominated by the death rate in hospitals. Instead, though, we see an initial underestimation of CFR, and this is due to the time lag in deaths. This, in my opinion, matches very well with the evolution of CFR that we saw in places like South Korea and Germany, where there was an initial very low CFR estimate that has been creeping up over time. I think both places had pretty good testing in place before the epidemic began to take off (South Korea more so than Germany, but I think both did pretty well).
This is an important context in order to understand the 0.37% CFR that Dr. Streeck recently reported. It needs to be understood that this number would correspond to a point on the red line on this graph: a point at which all deaths so far are known, and also all infections (statistically in this case due to a serological study). If you look at where Germany as a whole is on the curve at the time Dr. Streeck reported his conclusion, I think you will see that it matches in this scenario at a point in time a little past the 1/3rd mark--the point shortly after interventions are starting to flatten the curve.
This means we should not be surprised to see the CFR in Germany increase over time above Dr. Streeck's preliminary report. Doubling or even tripling would not surprise me.
This is an important context in order to understand the 0.37% CFR that Dr. Streeck recently reported. It needs to be understood that this number would correspond to a point on the red line on this graph: a point at which all deaths so far are known, and also all infections (statistically in this case due to a serological study). If you look at where Germany as a whole is on the curve at the time Dr. Streeck reported his conclusion, I think you will see that it matches in this scenario at a point in time a little past the 1/3rd mark--the point shortly after interventions are starting to flatten the curve.
This means we should not be surprised to see the CFR in Germany increase over time above Dr. Streeck's preliminary report. Doubling or even tripling would not surprise me.
Conclusion
Attempting to evaluate the CFR of a disease while it is in mid-progression is fraught with problems. I have demonstrated only two of the problems with this very over-simplified model. Therefore, the best projections of disease fatality do not use this kind of simplistic logic. If you want to see the more sophisticated way in which these things are done, I encourage you to look at the disease severity study which the Imperial College study used, which I discussed in this blog post earlier: https://darkenedintellect.blogspot.com/2020/03/the-imperial-college-study-part-3a.html .
No comments:
Post a Comment